Language is a beautiful, ever-changing form of communication, and technology can reduce the problem of low-use languages available on the internet.
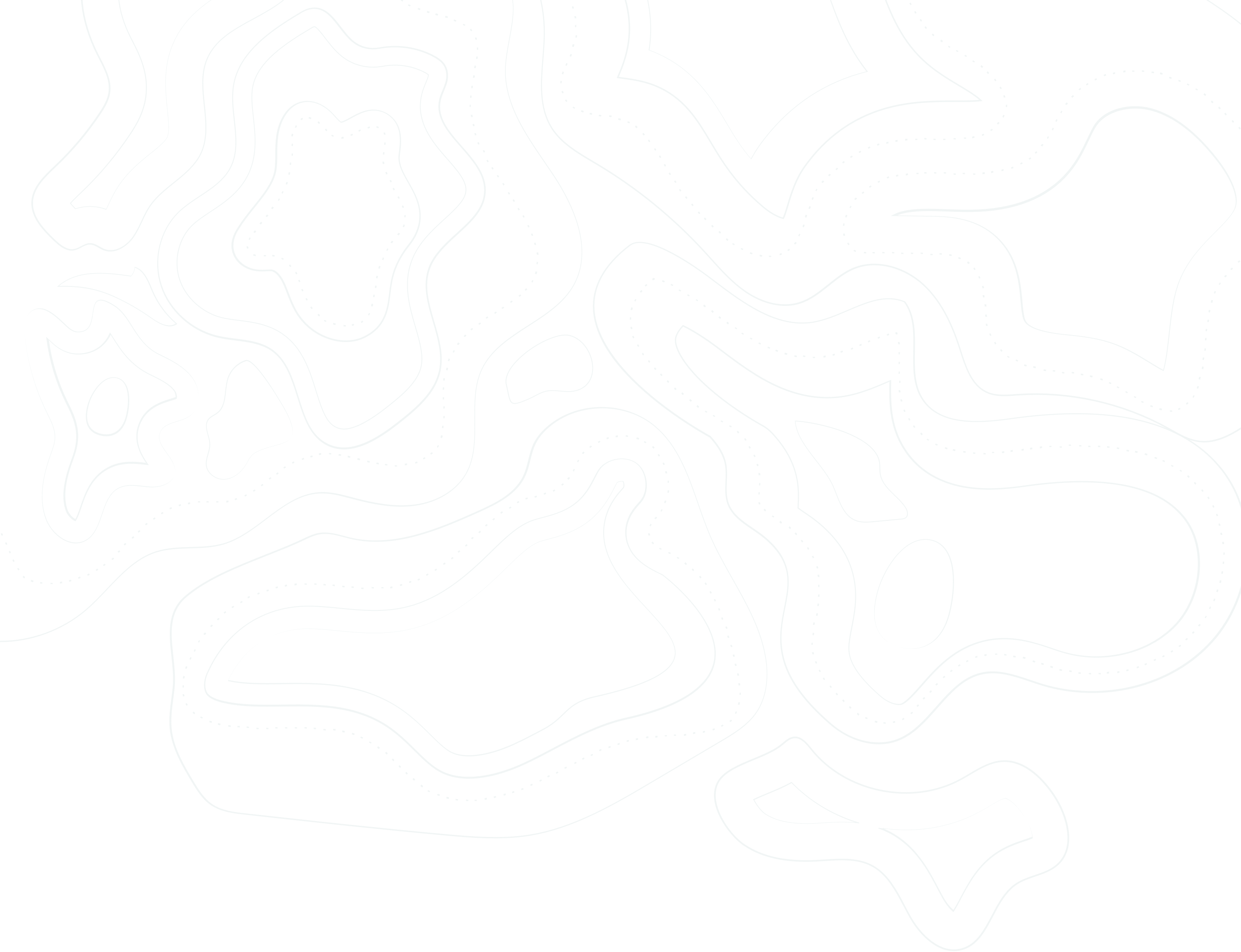
404 Language Not Found: Improving Low Resource Language Use in AI
Article Mar 19, 2021
David Anderson
You found this article! Great job! Thanks to Google, a couple of links, and terabytes of data on the English language, the computer found what you wanted. However, if you spoke the local African language Bantu, good luck getting Google or Amazon Alexa to work for you.
The internet has a blind spot: if few people use a particular language online, those messages mean nothing to AI or virtual translation apps. In other words, this blind spot includes any native speaker of a local dialect with no second language fluency. Think this is a minor problem?
In large parts of Africa, India, and South America, the spoken dialects have little virtual existence. They’re missing:
- A large amount of text (books, social media posts, blogs)
- Any language formalities (dictionaries, thesauri)
- Task-related uses of the language (text-to-speech, Google Translate)
With a low amount of these essential language resources, a typical AI function like Gmail’s spam filters or Apple’s Siri will struggle to
perform well. AI personal assistants use resources in their natural language processing (NLP) systems to understand text, so with a language
like Azeri spoken in Azerbaijan, Google Translate looks even more broken than it already is. Exciting creations in new languages resources
are trying to close this technology gap, but not without obstacles.
Translator and Computer Programmer Needed
The difficulty with incorporating a low resource language is overcoming the two main obstacles: a translator and a highly skilled programmer fluent in the language. However, many low resource languages are local dialects with little technological advances in their lifestyle. You may find a translator, but a speaker with the training to code the language into the software? There’s often no one capable enough.
Governments of diverse countries like Brazil and India need a solution the most. Regulations and other mandatory communication with indigenous peoples remain incomplete without a computer-friendly translation. They’ve got to print the tax code in the native languages somehow.
Stuck on Translation
Finding native speakers helps start the process, but translating speakers is the proper first step. Complicated tasks like speech analysis, syntactic understanding, and contextual reasoning must combine with a reverse translation process to be successful. Even when you have ample language resources, translation efforts only worked in a single direction (English to Malayalam, and not vice versa), leaving the translation fatally flawed.
Secondly, all computers have a language they’re most familiar with: English. Compared to the low resource languages needing NLP translation, it’s an oddball, rarely proving whether the translation is successful for any other languages. “For vocabulary, word-order, morphology, standardized of spelling, and more, English is an outlier, telling little about how well a result applies to the 95% of the worlds communications that are in other languages,” says Robert Munro, a lead researcher on overcoming this digital language problem.
Solution in Progress
In the past, language translation was approached as a single comprehensive project. Texts in the language were compiled or drawn up, native speakers were consulted, and translation software was created for that specific language. However, this process is long, expensive, and sometimes wholly unfruitful; missing information leads to incomplete projects.
The traditional approach was successful with several low resource languages like Punjabi, but recently, an approach called “transfer learning” is receiving attention as a possibly quicker and more successful option. In this method, computer models are trained to predict language patterns by using parallel languages to the target dialect or, sometimes, a completely different set. When the model is refined, it becomes a powerful assistant in adapting low resource languages for the web. Another method called “polygot learning” creates a multilingual, universal language for computers to read that is more transferable than the typical English. Coding the target dialect into the universal language makes instant AI understanding and removes the English language barrier.
Close, But Not Finished
The problem of missing internet languages only grows. How do you keep a people group from dying off linguistically if technology doesn’t include them? It may be impossible to prevent language extinction if experts in translation and AI don’t continue towards a solution. Language is a beautiful, ever-changing form of communication, and technology nears a solution to working in harmony with all its variations.
Looking for a guide on your journey?
Ready to explore how human-machine teaming can help to solve your complex problems? Let's talk. We're excited to hear your ideas and see where we can assist.
Let's Talk