Due to advances in Natural Language Processing (NLP), many firms worldwide are interested in leveraging its power. NLP has impacted the daily activities of many through the use of Alexa and Siri to perform simple tasks. In this article, we will look at the applications of NLP and tasks solvable using NLP.
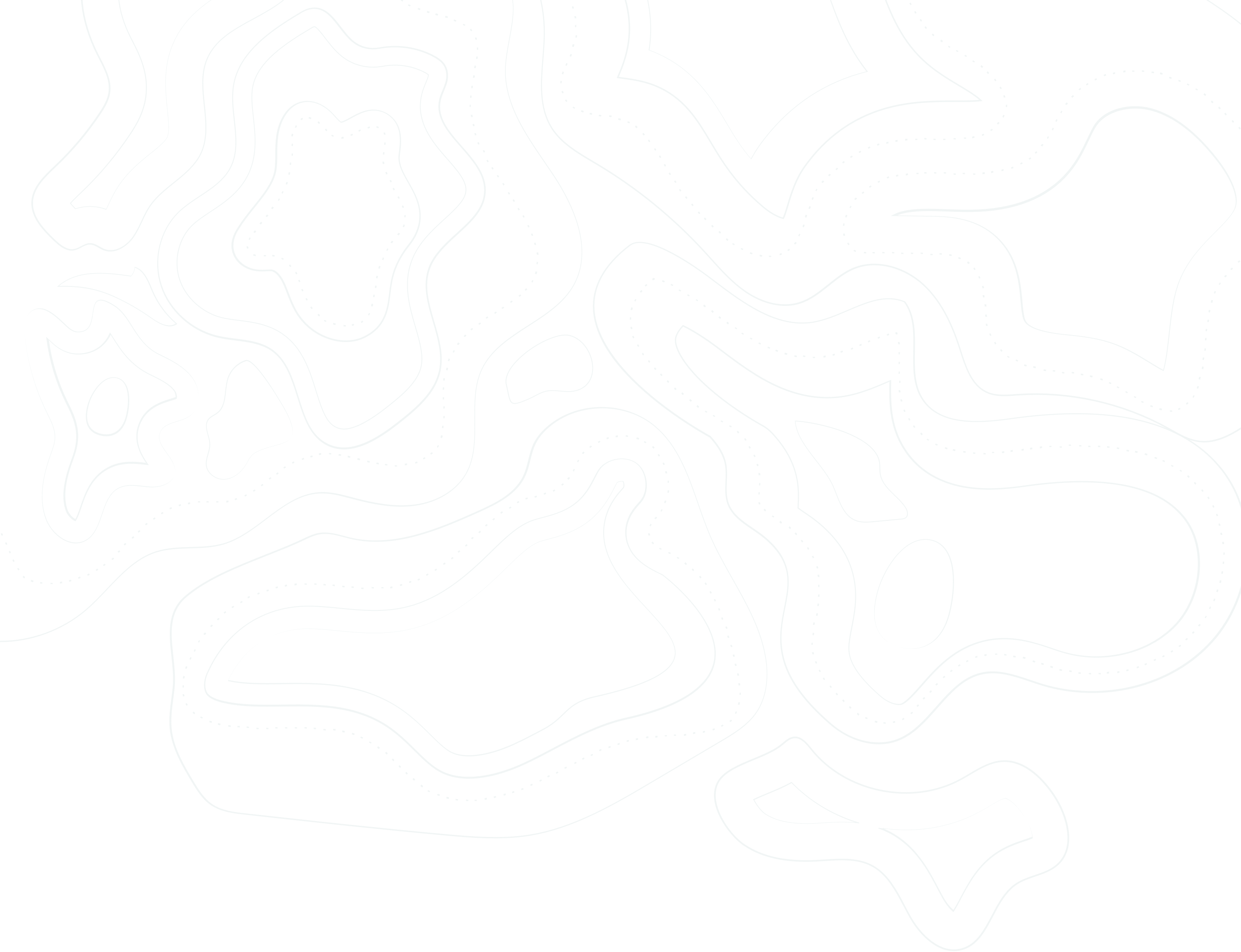
Applications of Natural Language Processing
Article Dec 17, 2020
Dave Anderson
Advances in Natural Language Processing (NLP) have led many firms to discover how to leverage its power. In this article, we will look at five applications of NLP as examples of emerging trends.
Voice Assistants
As mentioned, NLP enabled voice assistants have become commonplace with Siri from Apple, Alexa from Amazon, and Google Assistant. Voice assistants use NLP to recognize and transform spoken language into commands and provide an appropriate response. Voice assistants are useful as they enable us to complete many tasks on the go. For example, more and more people are using Alexa combined with smart home technology to set the thermostat, turn on lights, and even turn on their fireplace and warm a room.
Chatbots
NLP has matured enough for routine customer questions that many companies are beginning to deploy chatbots for customer service. Complex queries are automatically redirected to human customer care agents. This technology supports both the customer and the organization. For routine questions, customers do not have to wait for a reply. Organizations benefit by utilizing the customer care team for those interactions that require human engagement, rather than wasting their time with simple questions.
Text Analytics
The goal of text analytics algorithms is to provide a method to analyze non-structured data such as the notes in medical records or maintenance records. Companies with a large customer base may struggle to review customer feedback left on various platforms. By applying NLP techniques, it is easier for companies to address negative reviews. Text analytics may also provide insight into a product’s performance in the marketplace.
Recruiting
Human Resources departments at large organizations are often flooded with job candidates. Reviewing hundreds of resumes and applications can be time-consuming and tedious. NLP techniques extract key features regarding a candidate, such as skills, name, location, and education. These features are summarized to represent the candidates and then classified into fit or not-fit for a particular role.
It is vital for recruiters to be aware that these tools may bias against gender and minorities. Amazon shelved an NLP-based tool that showed a bias toward women. While these shortcomings lead organizations to be skeptical of such hiring tools, the early signs are promising.
Systems Biology
In a study on protein-nucleic acid interactions, systems biologists used protein sequence features defined by Position-Specific Scoring Matrix (PSSM). Unfortunately, PSSM contained insufficient evolutionary information on the binding sites of DNA and RNA. Support Vector Machines (SVMs) based NLP has increased the predictability of sites for DNA and RNA-binding. Further improvements in accuracy were demonstrated when both PSSM and SVMs work together.
More NLP in the Future
There are many applications of NLP, and new applications are deployed daily. NLP has made small tasks very easy to complete, and we can only imagine its potential in the future. If you are curious about applying NLP techniques to your unstructured or text-based data, please reach out.
Looking for a guide on your journey?
Ready to explore how human-machine teaming can help to solve your complex problems? Let's talk. We're excited to hear your ideas and see where we can assist.
Let's Talk