Applying Design Thinking can help us understand the human and the machine components of a data project to achieve the goals of a data science research project and deliver the highest value.
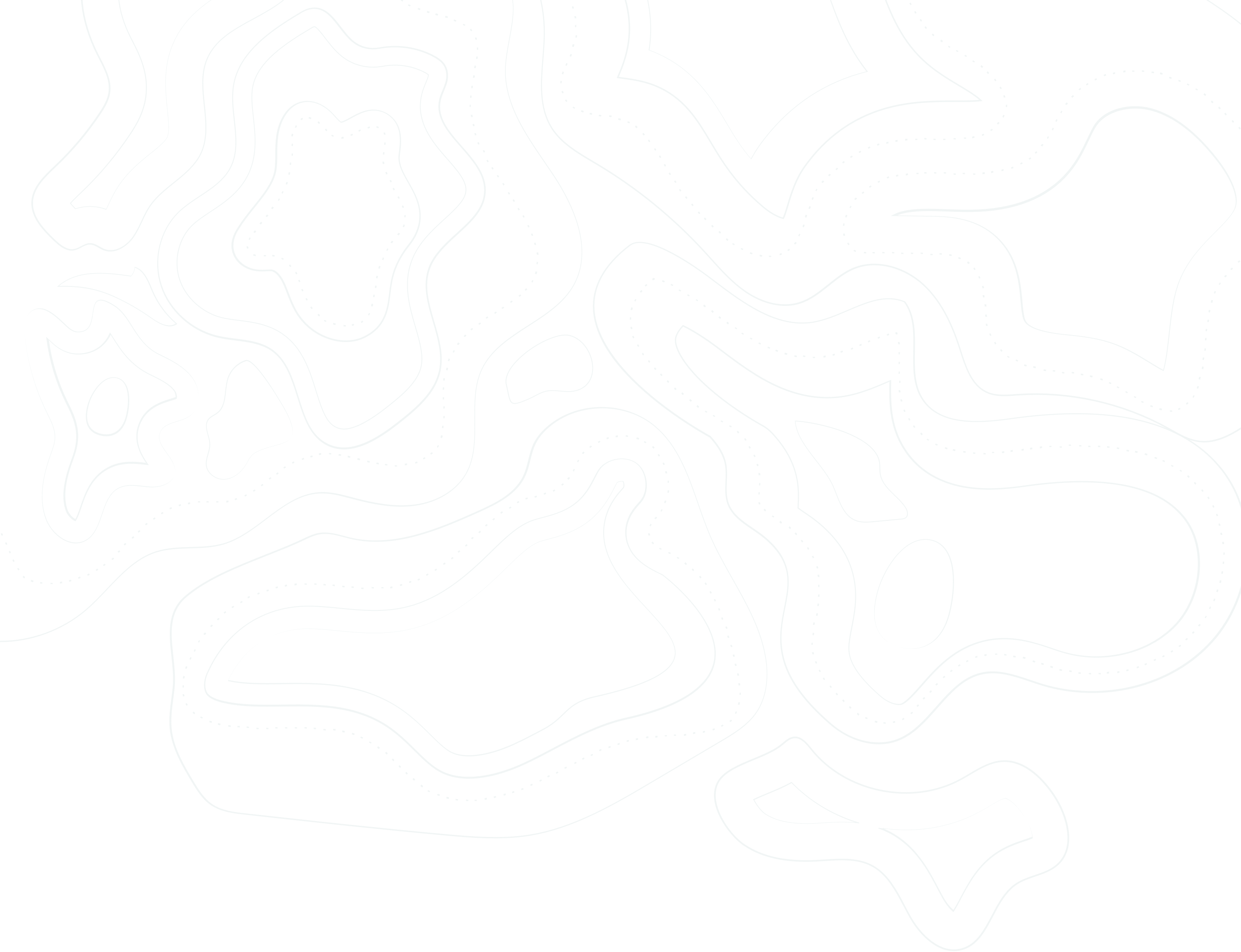
Augment Data Science With Design Thinking
Article Feb 25, 2022
Dave Anderson
Data science helps organizations leverage the vast amounts of available information today to derive timely insights and inform accurate decision-making. As this relatively young discipline matures, professionals seek ways to augment their practice and address shortcomings.
Challenges in the Data Science Research Process
A data science research process consists of five key steps. They involve framing the problem, collecting the data, exploring the data, performing in-depth analysis or building models, and communicating the results. Ultimately, we aim to ask the right questions that lead to relevant and actionable insights.
Framing a problem involves stating a hypothesis and developing a strategy to answer a question. In the past, the data available often constrained the questions we asked. The rise of big data has essentially eliminated this limitation—allowing us to start with a strategic question or direction when making a hypothesis. Yet, most data scientists have not evolved their approach.
The mindset of posing a question based on the available data is like putting the cart before the horse. An incomplete understanding of the problem can prevent us from asking the right question to uncover meaningful insights. If we can't frame the problem to answer the most strategically valuable questions, we won't get the highest return on the effort we spend in the next four steps.
Data scientists need to uncover meaningful insights from datasets during the data exploration process. Yet, many struggle to develop a deep understanding of the research questions and examine the data from different perspectives. This lack of knowledge often prevents them from going below the surface to derive valuable and relevant insights.
Another challenge can creep up during the analysis or model-building stage. We may become so engrossed in the data that we get sucked into a rabbit hole, losing sight of the project's primary objectives. This pitfall can lead us to produce an outcome that fails to answer the original question we set out to answer.
Then, data scientists have to turn the outcomes of the analytics process into relevant and actionable insights for the stakeholders. Failure to effectively communicate the findings means we may not justify the investment made in data scient research and analytics.
Lastly, we must ground the insights and outcomes in business results, which fundamentally revolves around human interactions with business processes and technologies. As such, data science must go beyond number-crunching to generate insights that embrace non-linear thinking, creativity, options for exploration, and a user-centric mindset.
Navigating these complex challenges isn't easy, and we can't fix them with new software. We need to change how we approach data science and analytics.
Applying Design Thinking to Data Science
Framing a problem and creating actionable results is also a critical component in the Design Thinking process, which can help us examine the data science research process through a different lens and explore alternatives.
What's Design Thinking?
Design Thinking is an iterative process that guides us to understand the users, question existing assumptions, and redefine problems. The approach helps us identify alternative strategies and solutions that may lie beneath the surface.
The process takes a solution-focused approach to problem-solving. It helps us develop a deep interest in the people whom the outcome (e.g., a product or service) aims to benefit. It helps us tackle hard-to-define problems and reframe them in a human-centric way.
Applying Design Thinking to Data Science Research
Here are the fundamental principles of the Design Thinking process and how they apply to data science:
Empathize With Stakeholders
Identify your audience, understand their needs, and keep them in mind throughout the research and analytics process. You can also build informal feedback sessions into the process to ensure that your hypothesis and insights are addressing their needs as the project evolves.
Validate with Prototype
Increase your understanding of the problem and align with stakeholders' needs by creating smaller-scale models (e.g., a subset of data.) A prototype helps you validate your approach before investing effort into analyzing data or building models for the entire scope of the project.
Ideate and Iterate
Challenge existing assumptions, question how you frame the problem, and explore innovative ways to answer the question at hand. This non-linear journey requires you to consider diverse viewpoints instead of being confined to the data you have currently available.
Visualize the Insights
Communicate the complex analysis, models, and findings to stakeholders in a way that they can act on to create meaningful outcomes. Also, use visual means (e.g., diagrams, charts) to help a non-technical audience understand the process and insights.
A New Data Science Process Supported By Design Thinking
Here's how to incorporate Design Thinking into your data science research process:
Ideation
Design Thinking is a strategic approach that balances technical feasibility, desirability, and business viability. It helps us consider the users' perspective when framing the problem to ask questions pertinent to the stakeholders and their challenges.
You can employ the design thinking mindset to explore the problem and determine your hypothesis. You can conduct informational interviews with stakeholders, diagram the processes to test your understanding, and gather unstructured data to see the issue from various perspectives.
Exploratory Analytics
It can be overwhelming to organize all the input from the ideation phase. Design Thinking can help us make sense of critical learnings and identify areas to further investigate by creating process diagrams and frameworks.
Design Thinking can also encourage us to consider the input from both a qualitative and quantitative perspective, so we're not confined to the data we have available while keeping the needs and challenges of the stakeholder front and center.
Modeling and Prototyping
Design Thinking uses prototyping to quickly iterate ideas and get targeted feedback from stakeholders to explore different questions and possible solutions. These prototypes can help challenge preconceptions and biases while infusing more creativity into the process.
You can validate your approach every step of the way to ensure that the project's outcomes are relevant to the stakeholders. You can also glean insights into communicating the results, so they're relevant and actionable to your audience.
Presentation
At this point, you probably have more data and insights than needed by your audience. Design Thinking helps you select the proper information for presentation. This approach can also help frame the outcomes to communicate why the findings matter, how you reach them, and how stakeholders can take action to generate meaningful results.
Use descriptive analysis to present a finding or recommendation before explaining the process that led to the conclusion. Define the purpose before creating graphs, charts, and diagrams to ensure that the visualization can help you connect the dots and tell a coherent story about the data.
Final Thoughts
Applying Design Thinking can help us take a human-centered approach to data science. It guides us to prioritize stakeholders' needs, ask the right questions, and produce actionable outcomes. It also helps us consider both the qualitative and quantitative aspects of data analytics—the human and the machine components to achieve the goals of a data science research project and deliver the highest value.
Looking for a guide on your journey?
Ready to explore how human-machine teaming can help to solve your complex problems? Let's talk. We're excited to hear your ideas and see where we can assist.
Let's Talk