The combination of AI / analytics with proper UX can lead to a trusting user experience.
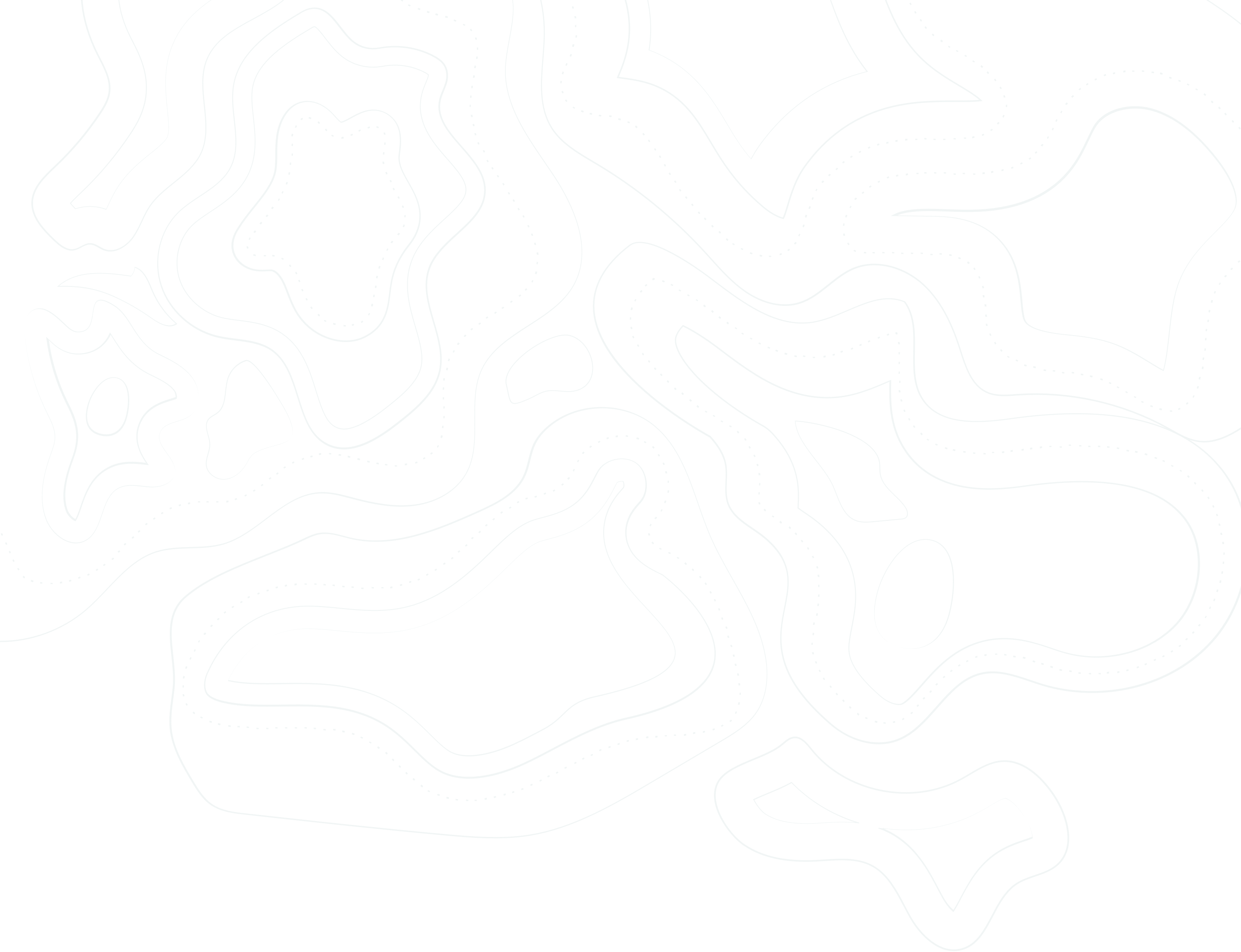
Computer as Partner: It’s All About Trust
Article Feb 05, 2017
Rob Keefer
We recently worked on a project that focused on the replacement of a medical case management system. Early in our user research we used the System Usability Scale (SUS) to evaluate the existing system.
The SUS score of that system was 44.5 out of possible 100 (the average score is 68). The system was so poorly designed that during one user interview a nurse began to cry as she described the various tasks she was required to complete in order to track patients and document her interactions with them.
As the outdated system was slowly replaced with a new system that increasingly incorporated data analytics and artificial intelligence, the SUS scores improved as shown in the figure below. The synergistic use of AI and analytics led to the development of a richer experience, and the SUS scores improved by more than 100%, jumping to 93.
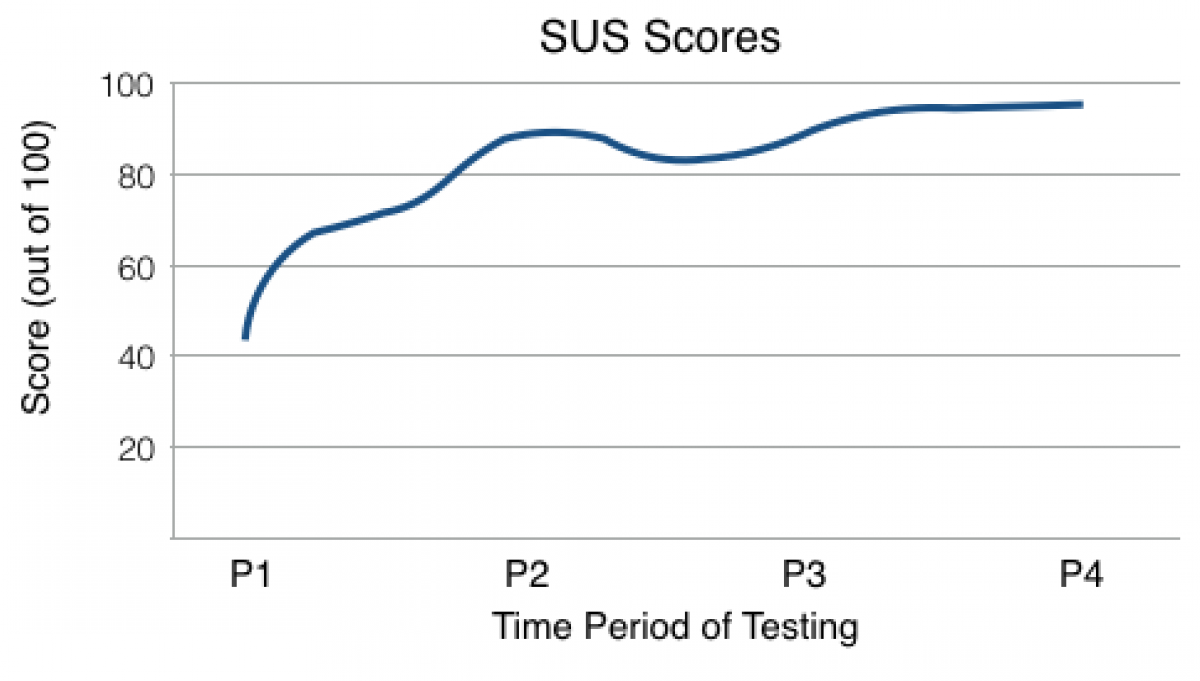
A time consuming task that the nurses performed involved following up with “high-risk” patients every 90 days. These patients were very sick, with multiple morbidities such as diabetes, cardio-vascular disease (CVD), and obesity. Believe it or not, many of these patients were also homeless, or transient, and used prepaid cell phones. When the prepaid phone plan terminated, the patient often discarded the phone (with its number) and purchased a new phone, with a new number, at a later time. This made it difficult for the nurses to locate and follow up with these patients. The nurses spent hours combing through patient records, medical insurance claims, and other data sources attempting to find contact information for the patient.
To support the nurses in this task, we created a feature called “Last Known Sighting.” For this feature we collated data from multiple sources: claims data, electronic health record (EHR) data, geolocation of emergency rooms, doctors offices, and other clinical locations. We added the geolocation of previous residences, such as friends and family homes, and shelters that the patient regularly resided. This data was used with few AI algorithms to compile a list of people/locations likely to know the whereabouts of the patient. However, rather than sorting the list of names and locations based on the data, we alphabetized the list.
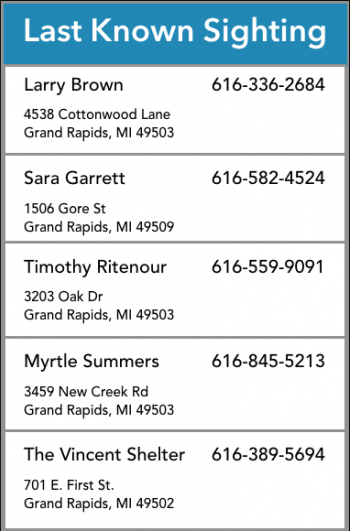
The list was alphabetized in order to engender trust. Often a data scientist displays information to users based on the data believing that transparency should rule the day. While this may be true in some cases, too often the user does not know what to do with the information and therefore misinterprets it. In the example above, there is only a 75% chance that Timothy Ritenour may have some information on the patient’s location. If we present the list with Timothy at the top and the score next to it, the user may expect Timothy to be helpful, when in fact there is a 25% chance that he is not helpful at all.
Based on our initial testing and observation, we believe that it is better for the nurse to call Larry and Sara before calling Timothy because the alphabetized list matches her expectations. She understands that the list is a helpful starting point rather than calculated truth. It is likely that if the tool is found to be frequently unhelpful, the user would abandon the tool all together. Thus, we have found that it many applications of AI it is better to present the calculated information as a helpful advisor may present it, rather than as an untrusted expert.
Based on the timing of the feature release and the uptick in user satisfaction, it seems that in this case the use of AI and analytics led to a trusting user experience, and played an important role in attaining a SUS score of 93.
Looking for a guide on your journey?
Ready to explore how human-machine teaming can help to solve your complex problems? Let's talk. We're excited to hear your ideas and see where we can assist.
Let's Talk