How can AI be exploited? There are a few weaknesses in deep learning systems that developers should keep in mind.
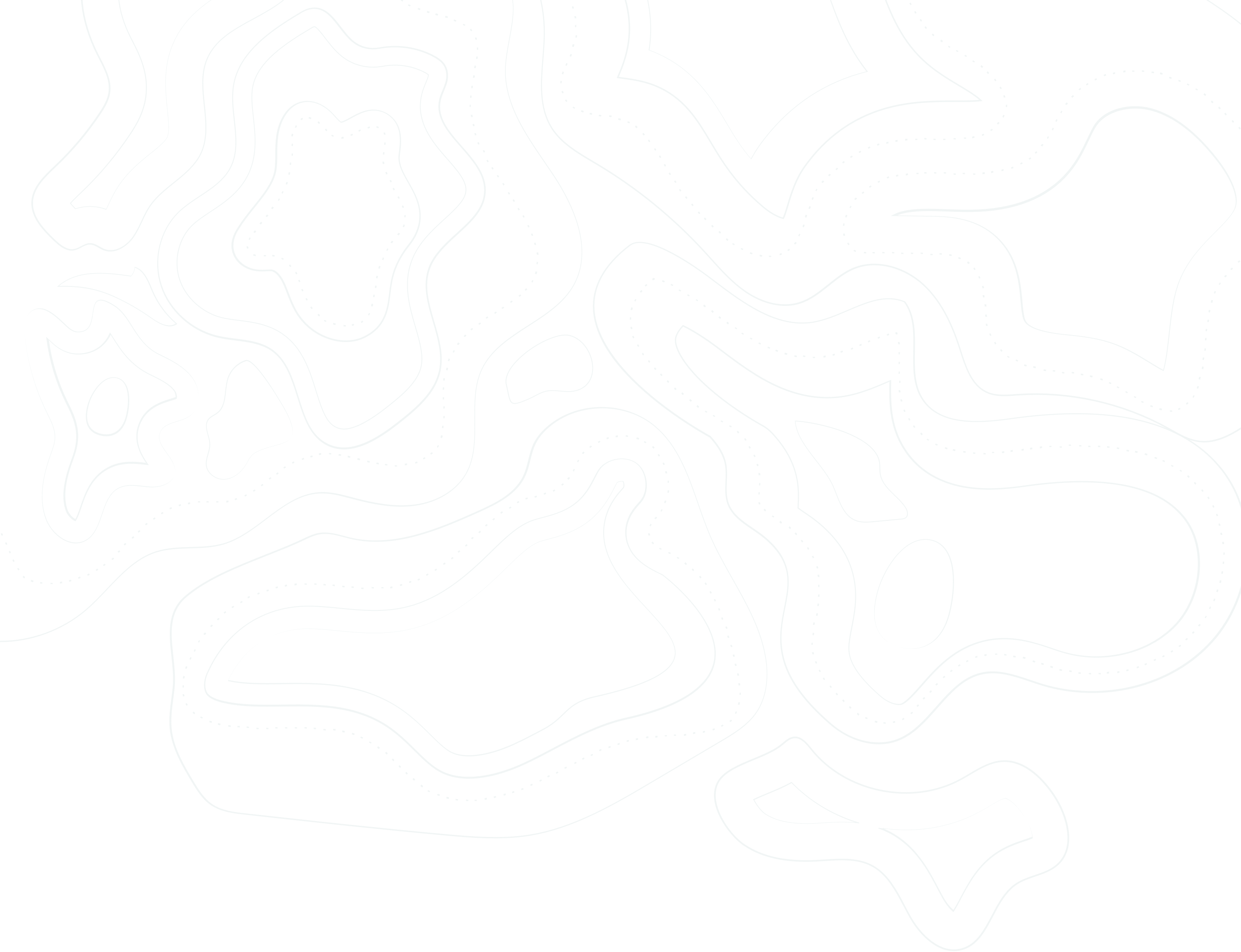
When Deep Learning Systems Go Wrong
Article May 09, 2023
Seth Tew
The day ChatGPT entered the online arena, global business took notice. An AI deep learning system like ChatGPT can translate languages, detect fraud, and diagnose medical conditions. Naturally, the release prompted various opinions on the tech, especially because similar deep learning systems exist in healthcare, finance, automotive, and many more industries. Amidst the users raving about deep learning system's benefits, it's easy to spot potential negatives and how AI could be exploited. Overall, there are a few weaknesses to deep learning systems.
The Cons Of Deep Learning AI
Lack Of Transparency
A deep learning system's complexity makes tracing its output's source almost impossible. Unlike traditional rule-based systems, where decisions originate in specific rules and logic, deep learning models use neural networks that are infinitely complicated, with millions or even billions of interconnected nodes producing linked results. With such a deep process, there's no way to know precisely how the system arrives at its conclusion. So little transparency makes holding the system accountable difficult, and the technological complexity leaves users and stakeholders in the dark about what creates the system outputs.
Can a deep learning model explain its predictions or results? Only sometimes, and not often in plain English. A multifaceted approach to computing like this makes the system skillful at creating but only sometimes understands what it has made. Explaining deep learning system reasoning becomes particularly problematic in industries where fairness and ethics are key, like healthcare and finance. With a reasoning process shrouded in mystery, this AI is tough to trust for critical problems.
Reliant On Models
Another challenge comes from AI's reliance on models. Deep learning systems build their computing on layers of models that handle different parts of the needed processing. Within these arises the issue of model bias. As systems are trained with introductory data, they'll develop preferences that lead to biased outcomes. For example, historical data that reflects societal biases could perpetuate those biases in AI's conclusions. In industries like criminal justice and human resources, the discrimination that could surface would make deep learning useless for those circumstances.
Network Attacks
The more technology is adopted, the more its weaknesses will be exploited, with attacks targeted at any vulnerabilities. Deep learning systems are most susceptible to using the limitations of their models against them, manipulating their inputs to mislead or deceive the system.
Even the slightest change to the data underlying the system's decisions could create faulty results or worse. For instance, a self-driving car's deep learning system could be fooled by placing misleading, confusing stickers on road signs, leading to dangerous consequences. Data attacks could undermine the reliability and robustness of deep learning systems and raise fears about what life-or-death decisions AI should avoid.
Look On The Bright Side
Though the consequences of corrupted AI are scary, nothing is set in stone. Deep learning systems consistently outperform conventional machine learning, which means the research and development of AI is far from over. Efforts are being made to make the inner workings of deep learning explainable and examine the models that make up their computing even deeper. As time goes on, the power of deep learning systems will feel like less of a liability and more like an essential assistant to your work.
Looking for a guide on your journey?
Ready to explore how human-machine teaming can help to solve your complex problems? Let's talk. We're excited to hear your ideas and see where we can assist.
Let's Talk